Fitting Into Your Company's Learning Cadence
How a data person might fit into your company's learning cadence, and what that means for company performance.
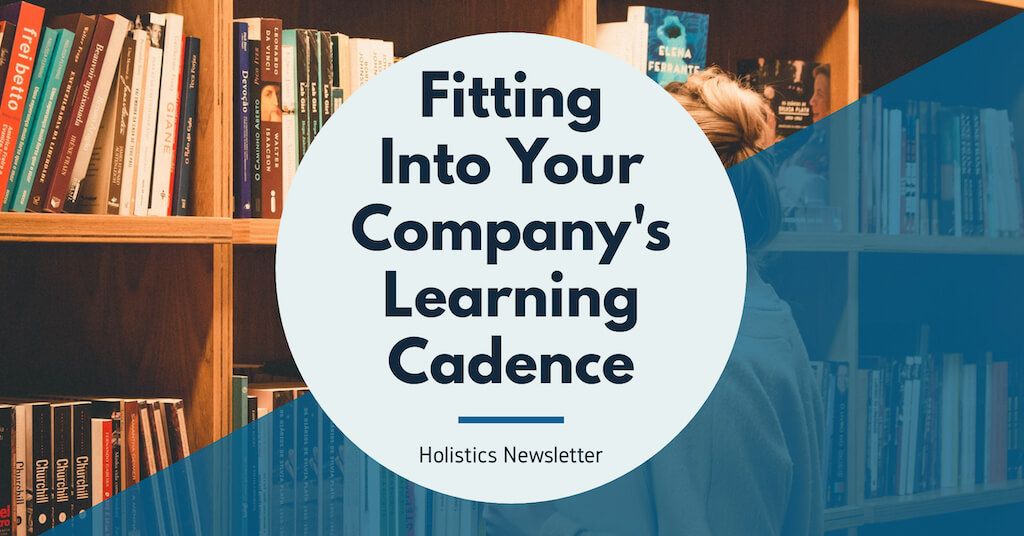
This newsletter was originally sent out on the 5th August 2021. Sign up here if you'd like more like this.
Inner Join is Holistics's weekly business intelligence newsletter. This week: fitting into your company's learning cadence, analytics engineers as purple people, and the difficulty of communicating changes with percentages.
Insights From Holistics
Fitting Into Your Company's Learning Cadence
One of the things that I've learnt from John Cutler recently is that every product team has a shipping cadence and a learning cadence:
Here's a little đź§µabout shipping and learning. Stick with me, because I'm not exactly sure where it will end up.
— John Cutler (@johncutlefish) June 28, 2021
Exhibit A: This company is shipping faster than it is learning. Which *may* not be a bad thing actually (more on that later).
1/n pic.twitter.com/l7biQQ3V9u
That is, a product team will rush to ship some software feature, but then they also must learn from the act of shipping. Did the feature do well? Is it used by many customers? Does the software help achieve some of the company's goals?
Cutler argues that things go well when you're shipping and learning at the same rate.
Things don't go as well when your shipping rate outpaces your learning rate. Then you're basically just chucking features over the wall without appropriate feedback.
How companies adjust to this are interesting. Some teams ship a lot, then pivot to learning (looking at data, combing through reports, etc), then go back to shipping.
Others slow their shipping rate down, in order to match their learning rate.
Some teams aren't very good at shipping, so their capacity to learn actually outstrips the rate at which they release new features and run new product experiments.
Bad teams charge forward, misinterpreting 'ship fast' as a directive to give up on learning.
Of course, good teams realise that shipping fast is a good thing — the company that moves faster tends to win. And at some point they realise that the only way to ship faster sustainably is to also learn faster — so they begin investing in better data tools, in better decision making culture, and in better build-ship-learn processes. They try to get their shipping and learning rates to line up.
Cutler's focus is on product teams, but really everything that he says applies to other parts of the company. Marketing has a ship/learn loop. As does sales. And bizdev. And ops.
Of course, some departments have more of a build-ship-learn workflow than others. But by and large, any sufficiently evolvable organization will experiment with things. It will test new processes, or marketing campaigns, or sales ideas. It will learn.
How is this relevant to data folks? Well, the learning loop in many of these sub-organizations depend on data, and therefore on the data team.
Cutler's thread is useful because it gives us an additional, systems-oriented tool to think about our companies. An 'organizational learning loop' now becomes a thing that you can reason about.
So here's a fun game — think about the various departments in your company.
How does the department learn? Who initiates changes to workflows? How did things end up the way they currently are?
How often does that department reach for data? Is the data they reach for good and easy to grok?
How fast are the people in it learning? Who asks the most for new reports? What types of reports do they consume?
Is there any way to change the shape of the demand? How can you help them increase their learning capability?
Of course, as part of the data team, you have little control over the department's experimentation culture or shipping speed. But then, as part of the data team, you're part of the solution to the 'how to learn faster' problem.
That's worth thinking about.
Insights From Elsewhere
​We the Purple People — dbt's Anna Filippova has a wonderful post on analytics engineers, with a neat little reference to Deloitte's Purple People: The Heart of Cognitive Systems Engineering:
This metaphor paints a world where humans with a deep understanding of business context in a particular domain are called red people ❤️. And humans with a breadth of technical expertise are called blue people 💙. Purple people 💜 are the people in between — they have a little bit of both that enables them to translate between red and blue.
I like this frame, and if you're reading this newsletter, I think you might, too.
​Communicating Changes with Percentages — Randy Au has a rigorous piece on the problems of communicating changes in data with percentages. His set of guiding heuristics are well-reasoned and sound:
- If the metric tends to move fairly steadily, either due to smoothing or is naturally low-noise, percent changes is rarely a problem. You can even plot the percent change curve if you want and it’ll highlight volatile points
- For noisy, volatile data that can’t be tamed with smoothing methods, showing percent changes comparing points in time can be useful for context, but plotting the historic percent change line tends to be less useful. Just directly discuss the trend if that’s of interest
- It’s rarely useful to use percent changes when the base number is a percent or ratio. It’s much easier to quote changes in percentage points or actual concrete numbers.
- If your audience is sophisticated, or you take the time to educate your audience, you can use log scales too
Recommended.
​What is the Right Level of Specialization? For Data Teams and Anyone Else — Eric Bernhardsson on a viral tweet of his:
I think this specialization of data teams into 99 different roles (data scientist, data engineer, analytics engineer, ML engineer etc) is generally a bad thing driven by the fact that tools are bad and too hard to use
— Erik Bernhardsson (@bernhardsson) July 21, 2021
Bernhardsson spends the rest of the article working through the idea that the over-specialization of roles in data team is probably due to bad tools; the implication is that he's building something cool to solve it. :-)
That's it for this week! If you enjoyed this newsletter, I'd be very appreciative if you forwarded it to a friend. And if you have any feedback for me, hit the reply button and shoot me an email — I'm always happy to hear from readers.
As always, I wish you a good week ahead,
Warmly,
Huy,
Co-founder, Holistics.
What's happening in the BI world?
Join 30k+ people to get insights from BI practitioners around the globe. In your inbox. Every week. Learn more
No spam, ever. We respect your email privacy. Unsubscribe anytime.