How to Restructure Data Teams Effectively, the Modern Analytics Leader, and the Evolution of Data Roles: An Interview with Christine Jiang
For this week’s episode of Designing Data Organizations, we spoke with Christine Jiang, former Director of Core Analytics at Vimeo, who was with the organization between 2020 and 2024.
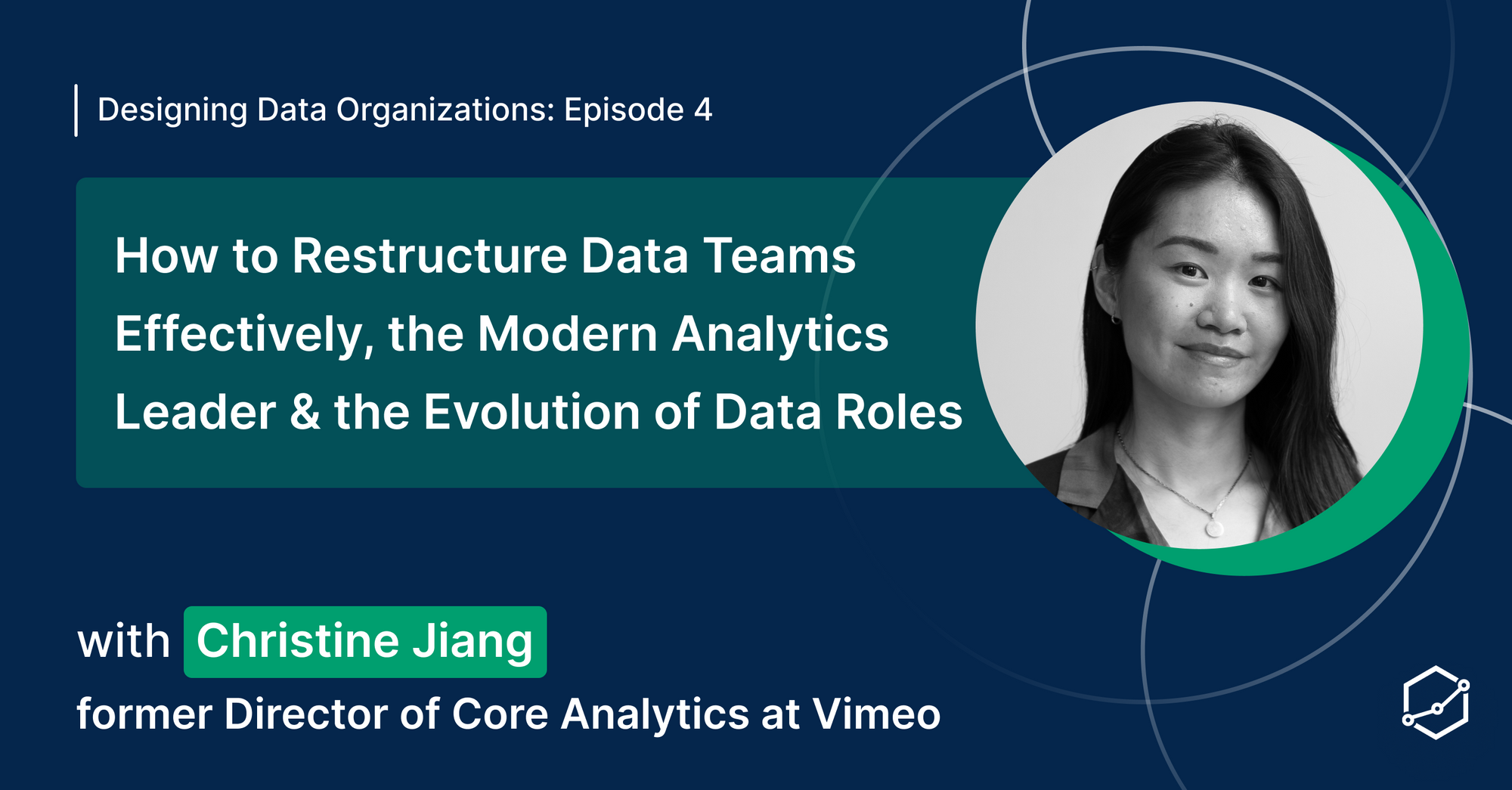
For this week’s episode of Designing Data Organizations, we spoke with Christine Jiang, former Director of Core Analytics at Vimeo, who was with the organization between 2020 and 2024.
Designing Data Organizations is brought to you by Holistics, where we delve into the intricate process of creating and optimizing data teams within organizations. In this article series, we sit down with seasoned data leaders from various industries to uncover their strategies, challenges, and success stories in designing effective data teams.
Founded in New York City in 2004, Vimeo provides a unified platform for users to create, collaborate, and communicate through video. The 1,200-strong company serves a diverse range of clientele including large enterprises, small businesses, creative professionals, marketers, and digital agencies. It was the first video-sharing platform to support high-definition content back in 2007. As of 2024, it has 300 million users and continues to focus on innovation and quality as a leading player in the video industry.
Christine is also the founder of the Analytics Accelerator, which is a mentorship program that transforms aspiring analysts to standout applicants by teaching them the skills used on the day-to-day job and equipping them with a winning job-hunt strategy.
• The analytics team at Vimeo was initially sliced by stakeholder verticals (product, marketing, etc.), but it was restructured to slice by data functionality.
• This reorganization had the advantage of allowing new teams like Core Analytics to focus on building cohesive shared data assets.
• To roll out data team reorganizations strategically, analytics should consider individual preferences for job types, timelines, and the relationship between data engineers and data analysts.
• Aligning data analysts to metrics is unconventional, but it incentivizes analysts to go deeper in understanding these numbers.
• The collaboration between analytics teams at Vimeo evolved along with the org restructure.
• As part of their inclusivity initiatives, Vimeo is accounting for personality fit for role suitability, which is especially vital for analytics leadership positions.
• Soft skills will be increasingly valued among data analysts, as more and more of the low-level work is handled by AI, leaving analysts to focus on higher-level work that requires more collaboration and strategic thinking.
• The traditional distinctions between data analysts, data engineers, data scientists have blended significantly within the last decade, as data roles evolve at a rapid pace.
Core Analytics at Vimeo
Gabriel Zhang: Hi Christine, congratulations on launching the Analytics Accelerator. I can’t wait to see how your programme inspires a new generation of data analytics experts! But for now, I’m interested in hearing about your experience leading the Core Analytics team at Vimeo.
Christine: Thank you, I’m really excited about this project too! Sure, let’s get into Vimeo.
The whole company is about 1,200 people, of which about 2% - or 25 people - are made up of pure analytics specialists. Historically, we had a “classic” team architecture, whereby analytics was divided into stakeholder verticals such as marketing, product, enterprise sales, etc.
Each vertical consisted of up to five data analysts, all of which funneled into a VP of data who, in turn, reported to the Chief Product Officer. Within this structure, I served as director of core financial analytics for one and a half years.
However, one drawback of this setup is that it’s not easy for the leadership to get a comprehensive and holistic view of what’s happening across all the verticals. So the analytics function was restructured last year to slice by data functionality rather than by stakeholder verticals.
My own role too, was revamped to build up the Core Analytics team. Basically, we focused on analytics team enablement, including developing the central data infrastructure and tooling used by all the other analytics teams.
I can’t speak in detail about the other three pods after the reorganization, but they focused on business insights, data science, and product analytics respectively.
“But when your analytics organization is sliced by verticals like marketing, sales…
Who is going to build this single, cohesive source of truth unless you have a team dedicated to its development, progression, and adoption?”
I’ve spoken to a few other analytics leaders at orgs of a similar size, and they’ve mentioned similar reorg experiences in the past few years.
Yeah, I think this is something borne out of a need for more mature data teams as a startup grows. Once your data team exceeds six or seven people, what happens is that you’ll have multiple people reporting similar metrics on different dashboards and reports, but they’re all defined slightly differently, and the numbers don’t align.
I should point out that Vimeo IPO’ed in 2021. When you become a public company, there is a much higher level of scrutiny around data governance. That requires having a centralized semantic layer, renovating the underlying data architecture, creating AI-powered data libraries, and so on.
But when your analytics organization is sliced by verticals like marketing, sales… Who is going to build this single, cohesive source of truth unless you have a team dedicated to its development and maintenance?
Indeed. And I always think that the analytics team that works on central tooling gets the projects that are both the most fun and intellectually-challenging, since they pretty much build the backbone for the rest of the analytics function.
Yep. The Core Analytics team was a pretty cross-functional one, in the sense that my new stakeholders were no longer a specific group such as finance, but all the other data pod leaders.
Responsibility-wise, my role also became much more project management-oriented. To give you an example, one of our key projects was an AI data catalog. As we were building out a single-source-of-truth metrics layer to centralize and standardize our metric definitions and code, the project grew to four data engineers and five analysts, plus 1-2 other leads over the course of five months.
The team structure for the initiative had to be fluid, because we needed both specialists and generalists. To build the metrics layer, we needed people who brought their knowledge from certain domains, while the generalists would work on more mundane data product development work.
For months at a time, we’d have engineers and analysts who rotated between focusing on their respective pods and participating in this initiative, and I spent a great deal of time liaising with them to make sure that we built a cohesive product.
Restructuring Data Teams Effectively
While we’re on the topic of team restructuring, I’d love to ask: For the ICs (data engineers, data analysts) whose job scopes were affected, how did they react to the changes?
Frankly, I think there were a lot of mixed feelings. However, I’d add that they weren’t always a reaction to the changes within the data team itself, but also the consequence of a macro environment that’s been experiencing a lot of turbulence and uncertainty lately.
Nevertheless, it can definitely come across as a disappointment to individuals who were, say, settled in a certain specialization that they excelled in, but are suddenly shifted into a function that might not seem as exciting.
On the bright side, it was also a welcomed change for some other people. For example, for some of the folks who were moved into data science, that’s a positive development for their career trajectory as the previous setup allocated very little space for data science work since there wasn’t a dedicated DS team. Post-restructuring, the goal was that they would be able to work on data science projects with goals that were distinct from those of general business intelligence.
Thanks a lot for answering that. The reason I asked is because, like you said, the macro environment is pretty rocky at the moment. I imagine that a lot of analytics leaders have had to deal with the psychological consequences of these changes, and the resentment that sometimes seeps through from people whose roles were affected negatively.
Do you have any personal thoughts or recommendations on how to roll out analytics reorganizations in an effective manner?
Well, thoughtful leaders of course aim to have a deeper understanding of how the day-to-day work experience of every individual in the organization will be affected by any proposed team restructuring.
Quite often, when organizational decisions are made at a higher level, a lot of things fall through the cracks. An example would be the designation of new team leads.
In a small to medium-sized company where working styles are really fluid, management sometimes takes it for granted that if someone’s really good in a certain skill, or has a personality that gels across all teams, they’d want to assume the mantle of tech or team lead in a reorganization. But that’s just an assumption and doesn’t always reflect the reality of how people feel about their new roles.
Secondly, it’s important to think about the longer-term trajectory of the business before pushing through a reorganization. Sometimes, leaders intuit correctly that there are gaps within an organization, and they react quickly to address these gaps, without realizing that they may be a temporary problem.
For example, if a company is planning to move from being B2C to B2B-focused within the next 12 months, you know that the teams will need to be realigned eventually anyway. So even if there are issues with the current org design, it’s probably worth waiting until the big changes at the business model level have been implemented first before initiating a reorganization, instead of having multiple reorgs in quick succession within a year.
“Another thing I’d suggest to modern data teams is to align analysts to metrics…
…I know that this is unconventional, but there are advantages to doing this.”
Oh yeah, we all know of companies that are guilty of implementing reorg after reorg. That can’t be great for talent retention, or hiring.
Yeah. Another thing I’d suggest to modern data teams is to align analysts to metrics, instead of placing them by stakeholder verticals (marketing, finance, product, etc.). I know that this is unconventional, but there are advantages to doing this.
The first is that companies care more and more about their North Star KPIs these days, so it makes sense to structure their analytics teams to revolve around them. Secondly, it incentivizes analysts to work in a different way; it motivates them to conduct deeper dives into specific numbers, as opposed to a more pedestrian approach when their official job scope doesn’t go beyond general reporting.
That’s a very interesting idea. I’ve never seen it myself, but I love the idea of an analytics org structure that is spliced by metric families.
I’d also recommend that analytics leaders consider what kind of relationship they want to see between data engineering and data analytics, e.g. whether DE should sit with DA under one big data umbrella, or if they should belong to separate departments, because this significantly changes the effectiveness of the working relationship between the two groups.
I think this is less important in small startups since they’ll naturally work closely together. But when you grow into a medium-sized company, maintaining the cohesiveness of data - having universally defined metrics, streamlined data assets, one common source of truth, etc. - becomes quite challenging.
And you want the engineers and analysts to work as closely as possible even if they’re located in different departments. Like, you’d want the data engineers to be equally mindful of the business context, and share the same sense of urgency as the data analysts.
Would you also be willing to give us insights on the collaboration practices within the analytics org? I assumed that must have morphed along with the team org.
The collaboration style of the analytics org at Vimeo actually evolved significantly over the years. Back when we were a small team of five to ten analysts, we used to do team-wide sprint planning every two weeks, and we shared a single ticketing system.
When the analytics function grew into four distinct verticals, each vertical started to function as an independent data team. So while we would all use JIRA, each vertical had its own sprint plans, quarterly priorities, and stakeholder meetings of different cadences.
And then the way we’d come together, is that we’d conduct monthly demos, share wider, monthly meetings with the data engineering team, as well as periodic open working sessions where we’d whiteboard different ideas and long-term goals for the analytics organization as a whole.
But post-restructuring, we had to change our collaboration style again. If you think about it… if you have one pod that is focused on data science, and another on reporting, they’d be sharing several metrics and data marts. It made no sense to replicate them for each pod, so we needed data assets that are flexible and reusable by each pod, and building these things requires close collaboration.
From a project management standpoint, the intra-team communications varied quite a bit among the teams. For Core Analytics, we largely operated as an independent team, because while I’d liaise with the other team leads on prioritization planning, the day-to-day work of my team doesn’t really require detailed inputs from the other teams.
The other three teams - Business insights, Data science, and Product Analytics - on the other hand, operated with a matrix model, which meant they all touched on a little bit of the other teams’ aspects. Lastly, most of the Product Analytics team is based in Israel. Because of the time zone and geographical separation, their sprint planning and prioritization were a bit more separated from the rest of the analytics organization.
The Modern Analytics Leader
I didn’t know that Vimeo had an Israel office.
It’s because Vimeo acquired a company a few years ago that was based in Israel, and Product Analytics was shaped around that existing team. In fact, Vimeo’s data team was split at one point across five different time zones: India, Israel, New York, California, and Japan.
I’m glad the topic of geographically distributed teams came up. It’s something that I want to explore a bit further, given that more and more analytics organizations - well, companies - are operating in this manner.
Let’s take the case of the Product Analytics team being concentrated in Israel. That sort of homogeneity can be advantageous, in that they naturally share a similar communication style and other unspoken social code. But there's also a risk that they’d be culturally disengaged from the rest of the company.
I’m curious about your thoughts on balancing inclusivity and the needs of the company in a modern, distributed data team, especially when these things don’t always align.
It’s an interesting question, because in reality, we’re mostly remote, and that dilutes the gravity of inclusiveness compared to when everyone is in the office. For example, companies used to worry about how people invite their colleagues to lunch, but that’s not an issue when social interactions are limited to a screen.
Nevertheless, I do think there are ways to be inclusive even in an all-remote setup. I mentioned earlier that reorgs are sometimes implemented in a top-down manner, without accounting for people’s individual preferences.
I want to highlight that before I left Vimeo, one of the progressive changes that the new VP of Data is trying to implement is to account for not only people’s strengths and skill sets, but also their personalities when evaluating role changes.
“Data teams will function increasingly like product teams…and if you consider the personality of successful product managers, they’re typically people who have a knack for negotiation, orchestration, and thinking about how pieces fit together in a larger system.”
To give an example, some people have no issues dealing with really difficult stakeholders, while there are others who dislike the occasionally confrontational nature of stakeholder management, but they make fantastic individual, technical specialists.
I’d try to align people’s personalities with the direction that they want to grow in to. If someone aspires to go down the managerial path, they’d need to excel at getting buy-in, being diplomatic, demonstrating a bit of political correctness at the right time, and all these other social-savvy traits.
On the contrary, if you put someone who doesn’t have the appetite for all these things into a leadership position, it might significantly limit the team’s impact on the wider organization. So I do think it’s important to think about these things.
That’s fascinating. I don’t think I’ve ever heard someone explain the dilemma of placing the right people in analytics leadership so lucidly, even though I reckon it’s actually a pretty common problem in companies.
Right, when you reach a senior level, regardless of what team you’re leading - even if it’s a technical one - a large part of your work still boils down to people dynamics.
A good analytics leader would be able to intuit the ideal role or place for each person in their org, and that’s critical; if you can put someone on a track where they feel genuinely motivated, they’ll go much further than someone who’s really technically skilled, but isn’t motivated in their role.
A good analytics leader should also be able to effectively explain - and market to other teams - the value of the team’s work, which can have a huge impact on the analysts’ morale. I’ve worked in three or four companies now and saw firsthand how the Head of Analytics influences how well the data team is regarded within the company.
I’ve always thought that one of the conundrums that analytics people face, is that there are far fewer options for us at the mid-career stage.
For software engineers - especially really brilliant ones - their career development pathway is basically limitless. At the largest tech companies, you can literally ascend the rank of a Level 10 engineer, and earn over a million a year.
But analysts don’t have such luxuries. Their pathway typically hits a “Principal Data Analyst” ceiling even in big organizations. From that point onwards, the analyst has to choose whether they want to pivot into an adjacent function, or go into people management which, for better or for worse, many people simply do not have the temperament for.
I also think that with the way the analytics industry is moving - with AI taking over more and more of the low-level, manual work that used to be done by data analysts - soft skills will be increasingly valuable.
For instance, I think data teams will function increasingly like product teams; you’ve probably heard of the phrase, “treat data as a product”. And if you consider the personality of successful product managers, they’re typically people who have a knack for negotiation, orchestration, and thinking about how pieces fit together in a larger system.
The Evolution of Data Roles
Speaking of evolutions, you’ve been in this industry for a decade. What are some of the biggest changes that you’ve observed in the last 10 years, and how do you think they’ll continue changing?
I think one of the biggest changes that have happened in the last decade, is that the boundaries between all the classic data roles have blurred.
Even though people outside of the industry might ask, “What’s the difference between a data analyst, data scientist, and data engineer?” Internally, we knew there was a distinction between the roles; the data analysts did the reporting work, the data scientists worked on sexy data models, and the data engineers supplied the data to both groups.
But instead of being three different colors, now it looks like a gradient. Tools like dbt have birthed the engineer-analyst hybrid, while data analysts are increasingly valued for their ability to move upstream as well as downstream in the data creation process.
I’m also a fan of the data product manager concept. If you treat a collection of dashboards as a product, then you can think of a data product manager as someone who’s building an ecosystem.
A data PM has to understand how everything that happens on the business side connects to everything that happens on the data side. This requires a holistic perspective, as opposed to a traditional data analyst who merely focused on making some graphs in a myopic fashion, and was limited to Excel, SQL, and some visualization software.
And I actually think that this is a good thing. A lot of people are scared that AI will take data analyst jobs away, but what I think will actually happen, is that AI will just take away the bottom layer of mind-numbing querying and manual dashboarding work, and allow people to focus on higher value work, such as thinking about the overarching design of their data architecture and ecosystem.
I think you hit the nail on the dot about data analysts being increasingly valued for their ability to move upstream. In fact, I think the bar keeps getting so much higher every year.
Business analysts are the new data analysts. Data analysts are the new analytics engineers. Data engineers are the new platform software engineers…
Yeah, it’s going to be really interesting to see how the analytics industry evolves in the next few years. But I actually believe that even with the rapid adoption of new AI technologies, the analytics industry will largely look the same five years from now.
It will always take a bit of time to migrate data assets and get a large number of people to adopt new tools effectively.
“A lot of people are scared that AI will take data analyst jobs away, but what I think will actually happen, is that AI will just take away the bottom layer of mind-numbing querying and manual dashboarding work, and allow people to focus on higher value work…”
And at the end of the day, we’ll still need humans to tackle some manual work such as data cleaning, because that requires a semantic understanding that AI can never perform.
I think you hit the nail on the dot about data analysts being increasingly valued for their ability to move upstream. In fact, I think the bar keeps getting so much higher every year.
Business analysts are the new data analysts. Data analysts are the new analytics engineers. Data engineers are the new platform software engineers…
Exactly.
Another role that I’ve seen emerge in recent years is the Fractional CDO - a Chief Data Officer who only works on a part-time basis.
Yeah, it’s now possible to have a plug-and-chug data team where you have an experienced data practitioner who sets up the data warehouse, data pipelines, and dashboards. And if they’re done in a sustainable and scalable fashion, technically that person only needs to show up occasionally for fine-tuning and maintenance, right?
Yep. Christine, I’m so glad we had this conversation. It’s been a fun and eye-opening one for me.
Likewise, thanks again for having me!
What's happening in the BI world?
Join 30k+ people to get insights from BI practitioners around the globe. In your inbox. Every week. Learn more
No spam, ever. We respect your email privacy. Unsubscribe anytime.