Snowflake and the Data Lakehouse
A quick look at what Snowflake's been up to re: the Data Lakehouse, an inside look at Amazon's data-driven decision making, and how Airbnb customized Superset to fit their needs.
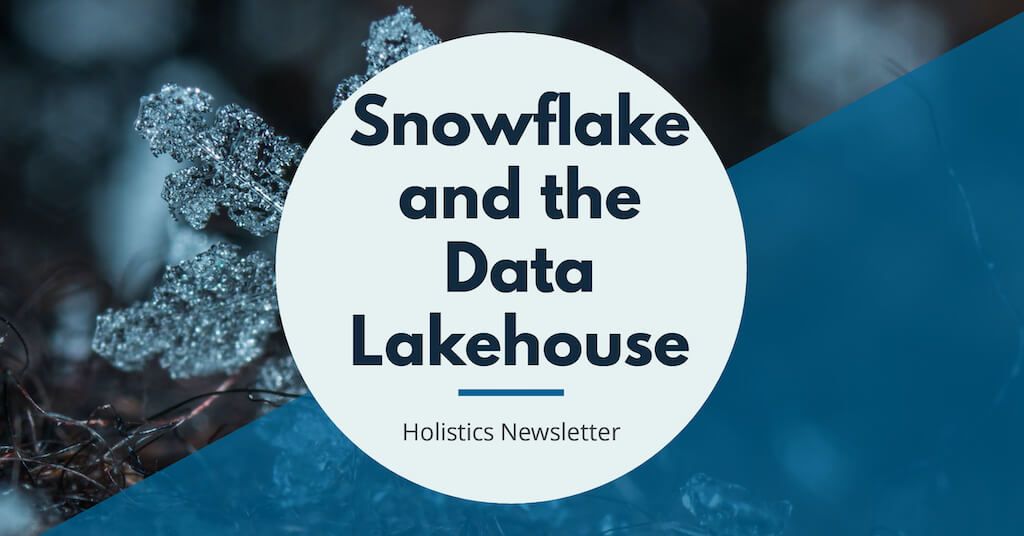
This newsletter was originally sent out on the 17 of February 2021. Sign up here if you'd like more like this.
Inner Join is Holistics's weekly business intelligence newsletter.
Happy Tet, or Chinese New Year, if you celebrate it! We were off last week due to the festive holidays in our part of the world.
This week: a quick look at what Snowflake's been up to re: the Data Lakehouse, an inside look at Amazon's data-driven decision making, and how Airbnb customised Superset to fit their needs.
Insights From Holistics
Snowflake's Data Science/Machine Learning Play
In the last edition of this newsletter, I wrote about how the 'data lakehouse' pattern seems to have become a thing. (For the uninitiated, this is the pitch that "hey, we can make your data lake as easy to use as a data warehouse, and, hell, you can start acting as if you have a warehouse inside your lake!")
I said this was a compelling pitch, and I gave you a bunch of links to podcasts, articles, and papers on Databricks's Delta Lake project, which proposes to do exactly that. I then said that we were watching this space, because it may or may not represent a change in the way data analytics is done.
The core value proposition is this: if you're just doing data analytics, your life is easy — you simply get a data warehouse and build around it. If you want to do data science and production machine learning, on the other hand, your life becomes very complicated, very fast. You'll likely have to maintain a warehouse for analytics, and then a data lake for unstructured data for ML, and then you'll have to build infra around both. The argument for a 'data lakehouse' is that you should be able to combine the two into one thing, and simplify your life.
That's the argument, at least. Would this be the case? I don't know — given my experience talking to more traditional businesses, I'm not convinced that every company needs to use ML. So there's probably some set of companies that would need a lakehouse desperately, and others that look at the whole thing and go "eh, I have problems getting my people to use data in their operations, and I can't even get quarterly numbers from Korea in a timely manner ... maybe later."
Here's where things get interesting, though. We know that Snowflake is an amazing data warehouse. And we know that companies are going to need an analytics capability before they need a data science or ML capability. So Snowflake has an interesting — if unproven — value proposition: use us as a data warehouse, they say, and then later, when you need to, you can use some of our more advanced features to do data science/ML.
This value prop is really new. In November last year, they announced a number of things:
- Snowpark — use an imperative programming language of your choice to execute workloads such as ETL/ELT, data preparation, and feature engineering inside Snowflake. (This is available in testing environments for now).
- Unstructured Data — dump your cat photos and videos into Snowflake, for machine learning purposes. (This is available in private preview for now).
- Row Access Policies — have all sorts of security constraints apply to returned result sets when queries are executed. Presumably this works for both data objects and workloads, so they apply to both Snowpark functions and the
cat videosunstructured data you've loaded into your lakehouse.
All of this is to say: Snowflake isn't taking the Databricks innovations lying down. If it works, it'll be a fascinating value proposition.
It is also, however, too early to tell how these things would turn out. Presumably you're going to need some way to load your unstructured data from Snowflake and pass them along to, say, Tensorflow. And then I presume you'll want to save the trained model back into Snowflake? We'll see. There's still a lot of tooling that needs to be built out.
If you're a data practitioner, just keep an ear out for developments in both projects. (Or, you know, stay subscribed to this newsletter — I'll tell you when there's something new).
Insights From Elsewhere
Identify Controllable Input Metrics — Working Backwards is a book by Colin Bryar and Bill Carr, about how Amazon really works ... by two people who were in the room when the techniques were invented. The book was published just last week, but I was waiting for years for someone to write something like it.
I want to highlight one big idea from this interview:
"Just think of a business as a process. It can be a complicated process, but essentially, it spits up outputs like revenue and profit, numbers of customers, and growth rates. To be a good operator, you can't just focus on those output metrics — you need to identify the controllable input metrics. A lot of people say that Amazon doesn't really care about profit or growth. I think that the data say otherwise, but what is true is that the main focus is on those input metrics," says Bryar.
"If you do the things you have control over right, it's going to yield the desired result in your output metrics. The best operators I've seen very clearly understand that if they push these buttons or turn these levers in the right way, they're going to get the results they want. They understand that process through and through."
These input metrics are usually customer-related. "Is the customer experience getting better this week than it was last week? That's harder to figure out than it sounds. So you monitor 10 or 20 different things, you experiment a little bit," says Bryar. "Measure them day in and day out — a great operator always instruments, so they know exactly what's happening. If you don't measure something, it's going to go wrong."
(...)
He shares some sample input metrics that Amazon closely monitors. "For the retail business, what are the prices down to the product level, compared to what's out there on the market? How many new items were added? How many are in stock and ready to ship via Amazon Prime? What is the average delivery time? What was the number of orders? How many promises did we miss? What was the number of defects?" he says.
"Another great input metric that doesn't see the light of day is that Amazon pays a huge amount of attention to inventory record accuracy. If I have an item, is it actually on the aisle bin and shelf that I say it's on? This is hugely important — if someone goes to pick that order and it's not there, you've missed that customer promise."
The bulk of these input metrics should describe how the customer used the product or service, Bryar notes. "It's like you're a sole proprietor in a coffee shop. You get to see how long the lines are. You get to see if people think the coffee's too hot. You have to recreate those metrics, especially as your business grows and there are more and more proxies between you and the customer."
This is gold. In the book, Bryar and Carr argue that unlike many other companies, Amazon's leaders are expected to dive deep and have a good grasp of all the input metrics in their business unit. They also explain that if anecdotes differ from metrics, Amazon's leaders are expected to tear the metrics apart (aka be skeptical of the metrics, not the anecdotes). And they describe a yearly planning process that Amazon calls 'OP1' and 'OP2', where the S-team (Bezos's top lieutenants) publish their overall goals, and then everyone submits specific, actionable goals with measurable input metrics that target those goals for the next year.
It is a fascinating look at what it means to be truly data driven company, at the one of the highest levels of operational excellence. If you want a summary of the business intelligence aspects of the book, stick around — we're working on a summary for you that should come out in the coming weeks.
How Airbnb Customised Apache Superset For Scale — Airbnb open sourced Superset in 2016, and two weeks ago, I linked to their announcement of reaching version 1. This post is a deep dive into all the ways Airbnb has customised Superset for their needs. This post is interesting because a) Airbnb remains the oldest in-production implementation of Superset, b) they're remarkably open about open sourcing tooling around it and finally c) you get a look at some of the thinking that went behind customising a BI implementation for scale. A good read.
In my last newsletter I linked to How to Make Dashboards Using a Product Thinking Approach by Shopify's Lin Taylor, but accidentally linked to Spotify's Designing Data Tools at Spotify. Oops! Thankfully, both are good links.
(Has anyone accidentally bought one company's stock, when they meant the other? Man.)
That's it for this week! If you enjoyed this newsletter, I'd be very appreciative if you forwarded it to a friend. And if you have any feedback for me, hit the reply button and shoot me an email — I'm always happy to hear from readers.
As always, I wish you a good week ahead,
Warmly,
Huy,
Co-founder, Holistics.
What's happening in the BI world?
Join 30k+ people to get insights from BI practitioners around the globe. In your inbox. Every week. Learn more
No spam, ever. We respect your email privacy. Unsubscribe anytime.